Understanding Bayesian Filtering: Enhancing IT Services and Security Systems
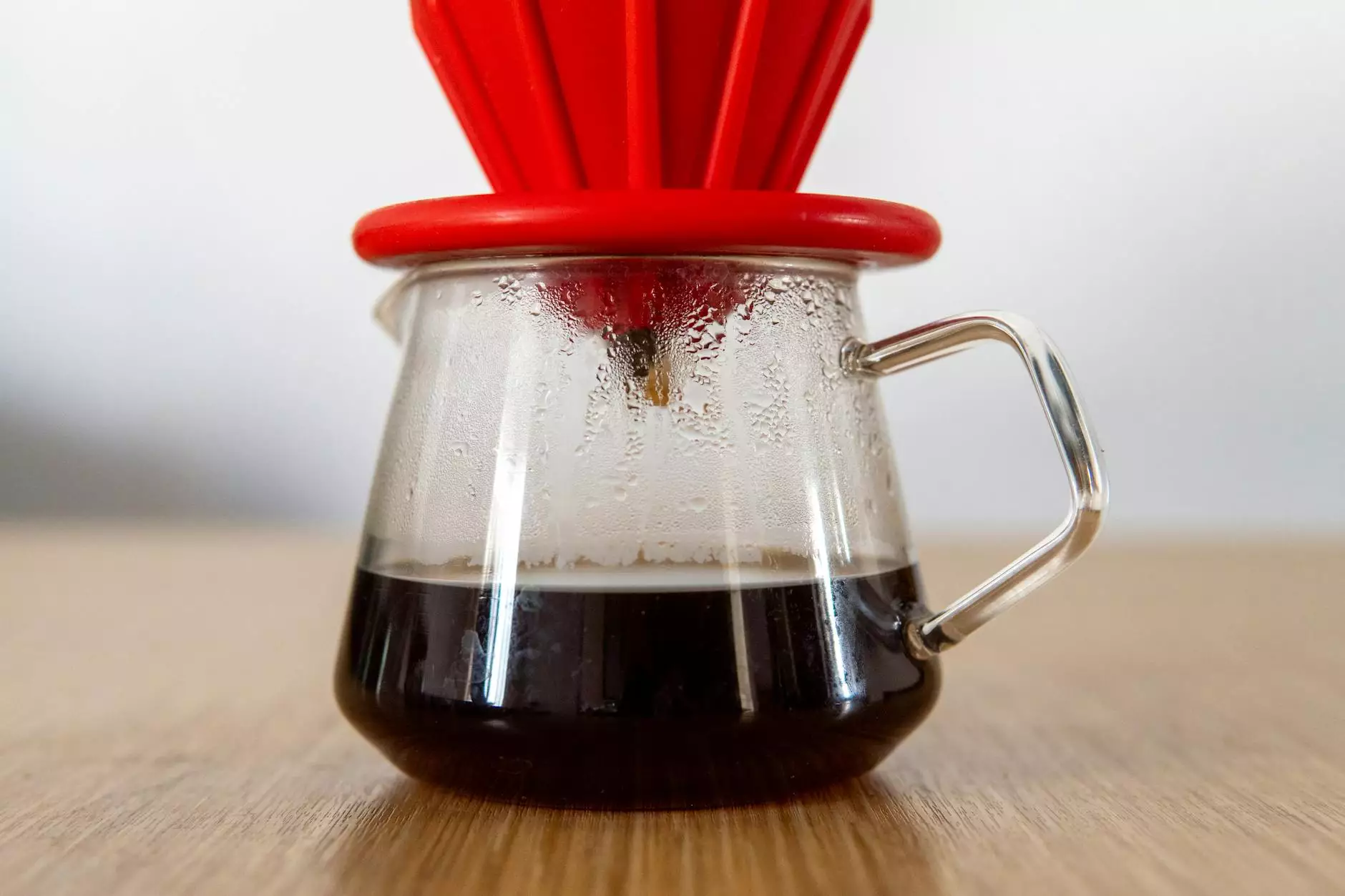
In today's ever-evolving digital landscape, businesses face significant challenges in managing vast amounts of data while ensuring security and efficiency. One remarkable technique that is often overlooked is Bayesian filtering. This powerful probabilistic method can transform an organization's approach to IT services and security systems. In this detailed article, we will explore what Bayesian filtering is, its applications, the benefits it brings to IT services and security systems, and how businesses like Spambrella can leverage it for operational excellence.
What is Bayesian Filtering?
At its core, Bayesian filtering is a statistical technique based on Bayes’ theorem, which is a mathematical formula that describes how to update the probability of a hypothesis based on new evidence. It’s widely utilized in various domains, particularly in spam detection, but its applications extend far beyond that.
How Does Bayesian Filtering Work?
The beauty of Bayesian filtering lies in its adaptability and learning capabilities. It works on the premise that each item—in this case, emails or network packets—can be categorized based on its features. Here’s a basic overview of how it operates:
- Training Phase: The filter is trained using a dataset of known examples of different categories (e.g., spam and non-spam). The algorithm analyzes these examples and calculates the probabilities of certain features appearing in each category.
- Calculating Probabilities: When a new item is received, Bayesian filtering calculates the probability that the item belongs to a particular category based on its features.
- Classification: Finally, based on the calculated probabilities, the filter classifies the item into the most likely category, making it a robust tool for decision-making.
Applications of Bayesian Filtering in IT Services
Bayesian filtering has widespread applications in the realm of IT services. Here are some of the notable ways it can be effectively utilized:
1. Spam Detection
The most common use of Bayesian filtering is in spam detection systems. By analyzing incoming emails and their features, businesses can significantly reduce the amount of junk email that reaches users’ inboxes. This not only improves productivity but also protects against potential phishing attacks.
2. Content Filtering
Organizations can implement Bayesian filtering to monitor and control access to specific types of content. For example, filtering out malicious files or unwanted content that may harm the organization’s IT infrastructure or compromise security.
3. Adaptive Learning Systems
Bayesian filters can enhance adaptive learning systems in various applications, enabling them to adjust and improve user experiences based on individual behaviors and preferences.
4. Predictive Analytics
Businesses can employ Bayesian techniques for predictive analytics in IT services, helping to foresee potential failures in systems or applications based on historical data trends. This can lead to proactive measures that can save time and costs.
Benefits of Bayesian Filtering in Security Systems
When it comes to security systems, the benefits of Bayesian filtering cannot be overstated. Here are some key advantages:
1. Enhanced Threat Detection
Bayesian filtering enables security systems to evaluate the risk associated with various network activities and detect anomalies. By constantly updating its understanding of what constitutes normal behavior, it can identify potential threats more proactively.
2. Improved Accuracy Over Time
Since Bayesian filters learn from new data, their accuracy improves over time. As more examples are processed, the system refines its predictions, leading to fewer false positives and false negatives.
3. Resource Efficiency
By effectively filtering out irrelevant data, organizations can allocate resources more efficiently. This means IT teams can focus on genuine threats rather than spending time sorting through benign data, enhancing overall productivity.
4. Flexibility and Scalability
Bayesian filtering is highly flexible and can be tailored to the specific security needs of a business. Whether it’s a small startup or a large corporation, the filtering system can scale accordingly, adapting to increasing amounts of data without a hitch.
Implementation of Bayesian Filtering in Business
For businesses considering implementing Bayesian filtering, particularly those in need of comprehensive IT services and robust security systems like Spambrella, the following steps can be instrumental:
1. Identify Objectives
Before implementation, businesses should determine specific objectives and outcomes they expect from the Bayesian filtering system. Whether it's better spam detection, improved security, or enhanced predictive capabilities, understanding the goal is crucial.
2. Data Collection
For effective training of the filters, businesses need to collect quality data. This dataset should represent the diverse scenarios the filter might encounter after deployment.
3. Training the Filter
Once the data is gathered, the next step is to train the filter. This involves feeding the data into the Bayesian algorithm, allowing it to analyze the features and learn how to categorize data efficiently.
4. Continuous Learning and Adaptation
After deployment, maintaining and fine-tuning the Bayesian filter is essential. The system should be monitored regularly, and its performance should be assessed. Continuous learning allows the filter to adapt to new patterns and trends.
5. User Feedback and Adjustments
Lastly, gathering feedback from users can provide insights into the system's performance. This feedback helps in refining the filters further, ensuring they meet the organizational requirements effectively.
Challenges and Considerations
While Bayesian filtering presents numerous advantages, there are also challenges to consider:
1. Quality of Data
The success of Bayesian filters heavily relies on the quality of data used for training. Poor or biased data can lead to inaccurate outcomes.
2. Complexity of Implementation
Implementing Bayesian filtering can be complex, particularly for organizations without substantial technical expertise. It may require investment in training or hiring specialized personnel.
3. Privacy Concerns
Handling large amounts of personal data raises privacy concerns. Businesses must ensure compliance with regulations and maintain transparency about how data is used.
The Future of Bayesian Filtering in Business
The future of Bayesian filtering looks promising as businesses increasingly rely on data-driven decision-making. As artificial intelligence and machine learning technologies advance, the capabilities of Bayesian filters will also expand, leading to more sophisticated applications across various sectors.
Organizations that adopt Bayesian filtering can expect not only improved operational efficiency but also heightened security and data handling capabilities. As threats evolve and the digital landscape becomes more complex, having a robust filtering system in place is not just an option but a necessity.
Conclusion
In conclusion, Bayesian filtering is a transformative tool for modern businesses, especially in the realms of IT services and security systems. By understanding its principles and effectively implementing it, organizations like Spambrella can harness its full potential, leading to better security, efficient operations, and ultimately, enhanced profitability. As we continue to navigate the digital age, leveraging technology like Bayesian filtering will be crucial in staying ahead of the competition.
Call to Action
If your business is looking to bolster its IT services and security systems, consider exploring the power of Bayesian filtering. Contact us today to learn how we can help you implement cutting-edge solutions tailored to your needs!